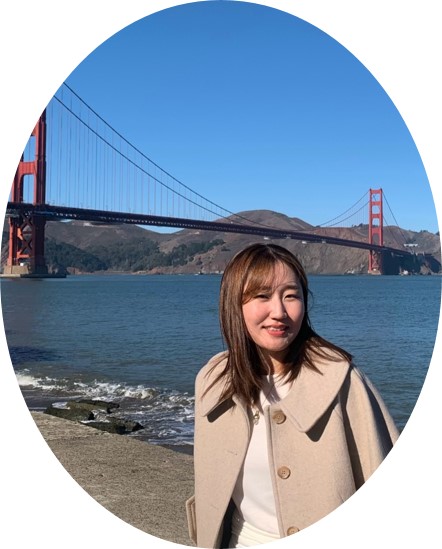
Yeaeun Kwon
Hello. I am a second-year Ph.D. student in Information Science at the University of Arizona. My research interests lie in Computational Social Science. Specifically, my research focuses on human and human interactions, social biases, and dynamics on social media by employing NLP techniques. Prior to my doctoral studies, I researched string pattern matching algorithms for my master's thesis, comparing short and long-term data. I received an MS in Computer Engineering from Korea Aerospace University and a BA in Economics from Hongik University.
Publications
-
CLULab-UofA at SemEVal-2024 Task 8: Detecting Machine-Generated Text Using Triplet-Loss-Trained Text Similarity and Text Classification [Poster]
Mohammadhossein Rezaei, Yeaeun Kwon, Reza Sanayei, Abhyuday Singh, Steven Bethard
Proceedings of the Fourteenth Workshop on Semantic Evaluation 2024 -
Developing a Text-Based Measure of Humility in Inquiry Using Computational Grounded Theory false
Sarah Bratt, Erin Leahey, Charles Gomez, Jina Lee, Yeaeun Kwon, Charles Lassiter
Proceedings of the Association for Information Science and Technology 2024
Research Experiences
-
International Influence in Science
This research investigates international influence in science through research articles. I am focusing on measuring influence between countries across time windows for 1995-2020, using text embeddings and similarity. The unique text embedding method I created represents the research characteristics of each country and enables to measure the influence between core and the peripheral countries in 5 research areas, which are Physical sciences and math, Medical Sciences, Technology and Engineering, Biological and Ecological Sciences, and Social and Behavioral Sciences.
Global Knowledge Lab, University of Arizona, Advisor- Charles Gomez -
Classification between human-written texts and machine-generated texts
The task of this project is the multi-class classification between human-written texts and five state-of-the-art machine-generated texts. I developed unsupervised learning and a triplet-loss-trained model using hard-positive and negative concepts.
Computational Language Understanding Lab, University of Arizona, Advisor- Steven Bethard